Calendar Icon
Nov 29, 2021
Person Bust Icon
By Karl Vogel
RSS
Submit a Story
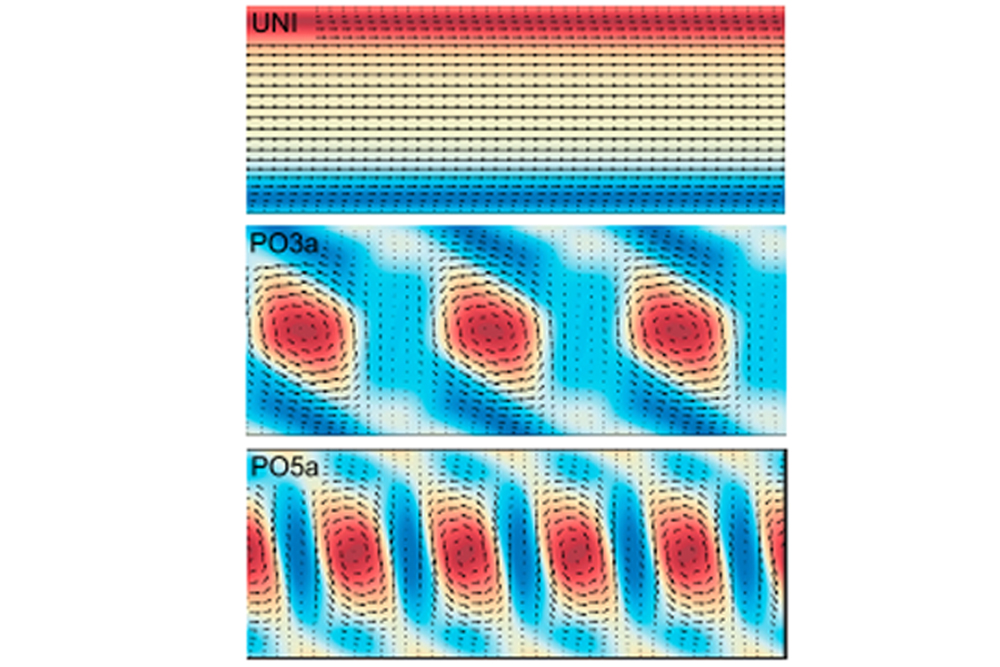
Taking lessons learned from animals who work in groups in the sky, on the ground and underwater, a team of Nebraska engineers is creating algorithms that can help individual agents in emerging technologies work together to improve our lives.
Piyush Grover and Jae Sung Park, assistant professors of mechanical and materials engineering, are creating algorithms to predict and control the collective behavior of these agents – such as robot swarms, a mixture of human-driven and autonomous vehicles on city streets, and micrometer-scale active matter.
And those algorithms are informed by what scientists have learned from birds, bees, pigs and fish.
"Scientists have known for centuries that you cannot come up with an understanding about the collective behavior of a group of agents by studying a single agent in isolation in great detail," said Grover.
Understanding how the patterns form in nature – like birds flying in V-shaped formations to conserve energy or fish swimming in schools to fend off predators – can have applications in these new technologies.
The first project – a three-year, $450,000 grant from the U.S. Department of Energy to Grover, Park and Mike Norton, a collaborator at Rochester Institute of Technology – is seeking ways to understand and control turbulence in flows of active matter, which is a dense collection of microscopic constituents such as cytoskeleton proteins or bacteria that consume energy to exert mechanical forces on their neighbors.
"Think of a collection of birds, where they are moving randomly in all directions. It's a disordered state" Grover said. "However, given a certain stimulus, such as the presence of a predator, the birds will flock and move in an orderly fashion."
Similarly, in microscopic active matter, the collection can start moving together spontaneously in the presence of enough energy input. However, this collective motion can become very complex and resemble fluid turbulence: chaotic motion of swirls as seen in the ocean and the atmosphere.
"With certain synthetic active matter, it turns out you can control the amount of energy input by shining light on it, and if you choose certain light patterns, you can control the flows and make the system more stable," Grover said.
Controlling these self-propelling flows, Grover said, can potentially lead to a new class of programmable materials, as well as novel liquid drug delivery systems for the human body. Park said these new configurations are especially interesting to the Department of Energy because of the many applications that it could create, such as producing new biofuels.
A key breakthrough in this work is development of a predictive model by breaking up the complicated motion of active fluid into its basic components known as Exact Coherent Structures (ECS). Examples of ECS include unidirectional flow or periodic patterns of ordered swirl motion.
The second project, funded with a three-year, $311,533 grant from the National Science Foundation to Grover, is looking to compute incentives that can make individual agents such as robots more likely to work together harmoniously with the capability to autonomously switch between different collective behaviors.
Grover is using Mean-Field-Game theory, a recently developed paradigm inspired by statistical physics for modeling rational agents.
With large robot swarms, often the objective is to control the collective motion and not the precise motion of each robot. Additionally, each robot may not have the power or capabilities to commiunicate with every other robot. Each robot can then be programmed to optimize its behavior based on some local information about its neighbors, maximizing the incentives or rewards that take into account the local information and some global state of the system.
"In nature this can be seen in schools of fish that often change their collective shape to evade a predator," Grover said. "We're looking to optimize these behaviors, for example, so swarms employed in surveillance, agriculture, atmospheric and ocean sciences, can do their jobs better."
Grover is also exploring similar ideas for congestion control in traffic consisting of a mixture of human-driven and autonomous vehicles.
Submit a Story